[ad_1]
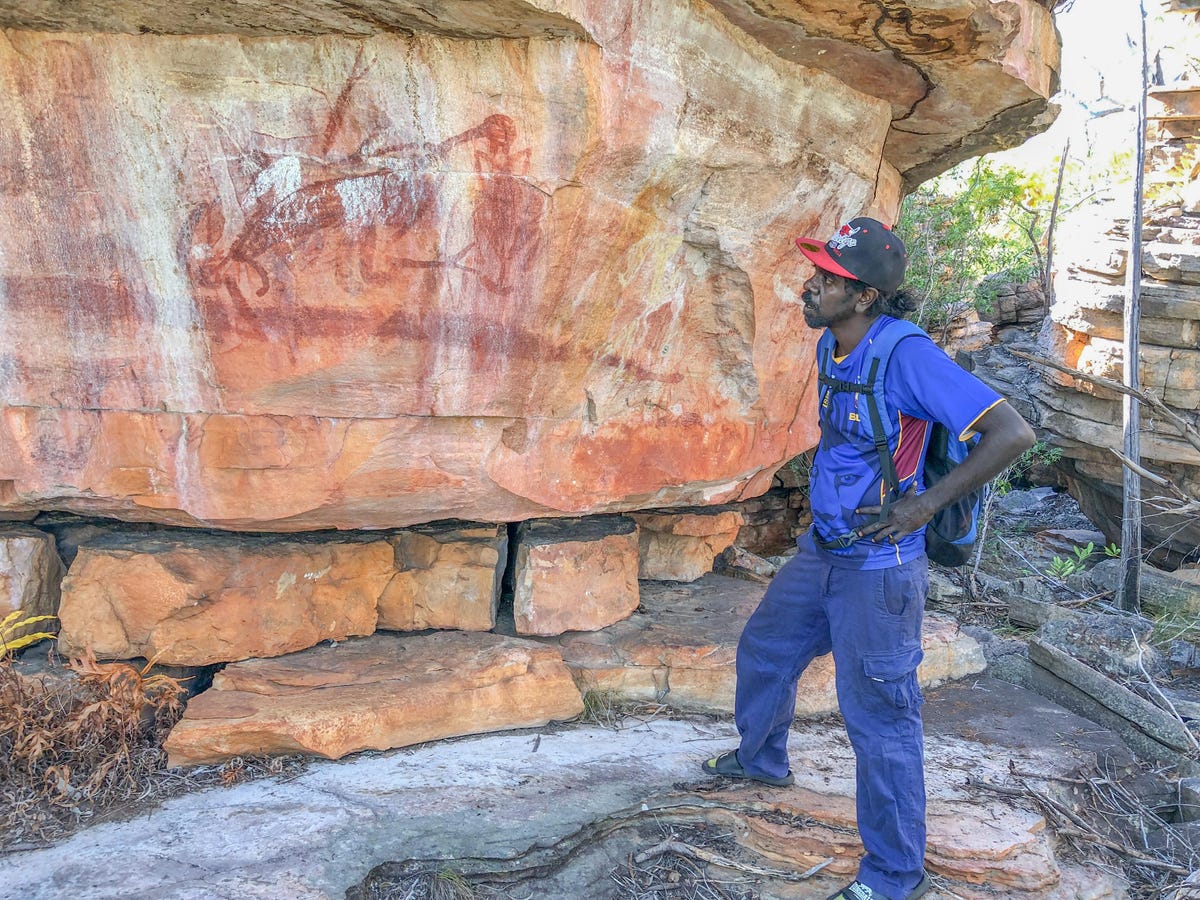
Young Dakal clan landowner Desmond Lindsay visits this site on his country for the first time. Most sites are remote and often impossible to even approach closely by vehicle.
Image: Mimal Land Management Aboriginal Corporation (MLMAC)
South Australian researchers have been working with the Mimal and Marrku traditional owners of the Wilton River area in Australia’s Top End to analyse the evolution of rock art through machine learning.
The study, led by Flinders University archaeologist Dr Daryl Wesley, saw the group test different styles of rock art of human figures in Arnhem Land labelled “northern running figures”, “dynamic figures”, “post dynamic figures”, and “simple figures with boomerangs” to understand how the styles relate to one another.
The team used machine learning to analyse images of rock art collected during surveys in Marrku country in 2018 and 2019.
The approach used previously trained and published convolutional neural network models and dataset combinations that were each designed and trained for object classification.
Co-authors of the findings published this week in Australian Archaeology, Flinders University PhD candidate in archaeology Jarrad Kowlessar and Dr Ian Moffat, told ZDNet the team then used transfer learning to deploy these networks on its dataset without retraining and then analysed the way the models responded or activated on a rock art dataset.
“This approach allows an unbiased classification of style as well as allowing us to make use of neural networks without access to a large sized dataset of rock art that would be required for training a model from scratch,” Moffat said.
“Our analysis of the model activation was conducted using the ‘t-distributed stochastic neighbour embedding (t-SNE)’ technique which is a non-linear method for dimensionality reduction. This method helps make sense and interrogate why the models have activated in the ways that they have for the different data points.”
The reconstructed rock art chronology uses existing datasets of more than 14 million different photos of animals such as dogs, cats, lizards, and insects to objects like chairs, tables, and cups.
“In total, the computer saw more than 1000 different types of objects and learned to tell the difference between them just by looking at photos of them,” Wesley added.
“The important skill this computer developed was a mathematical model that has the ability to tell how similar two different images are to one another.”
The methodology removed a large degree of individual human interpretation and possible bias by using a machine learning approach called transfer learning. This allowed the computer to understand how each style related to one another directly, independently of the researchers.
Moffat said the team began the research as it was excited by the possibility of using digital technologies to help understand Australia’s stunning rock art record.
“In particular, while traditional rock art analysis is a great way to record motifs, the interpretation of these figures relies on the researcher using their own judgement to classify images on the basis of style,” he said.
“Obviously the result of these classifications are heavily influenced by the researcher’s previous experience and training. Our new transfer learning approach works without being influenced by the biases of researchers and so provides an entirely new lens through which to understand style.”
Kowlessar said the algorithm ordered the styles in the same chronology that archaeologists have ordered them, by inspecting which appear on top of which.
“This shows that similarity and time are closely linked in the Arnhem Land rock art and that human figures drawn closer in time were more similar to one another than those drawn a long time apart,” he explained.
“The exciting thing about our research is not that it is finding things that humans have missed but that it is replicating the results of other studies that have used a more traditional approach,” Moffat told ZDNet. “This demonstrates that our approach is working and suggests it has exciting potential to contribute to rock art studies elsewhere.”
He said the method used can be applied to a variety of stylistic identification.
“This is very well suited to research in art where ‘style’ is a dominant factor but also applicable to other materials,” he said. “One such place we would like to continue to use this is in the analysis and identification of animal species in rock art.”
RELATED COVERAGE
[ad_2]
Source link